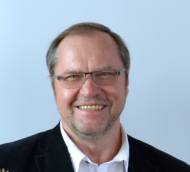
Ivars works for the EDI since 2020. His research interests have been relating to Explainable AI, Deep Learning, Computer Vision, and Neuroscience. Currently, his active research involves explaining AI in medicine, interpreting complex tasks by using deep neuron networks, and training them with supervised learning. The use of Explainable AI in medicine makes it possible to develop self-explainable medical systems that include visualization and language models. In turn, they can justify a decision for further medical treatment based on the interpreted generated diagnosis. Other research interests include understanding the operating principles of deep neuron networks, applying them to high-tech areas, such as the space industry and astronomy.
Recent projects
- Efficient module for automatic detection of people and vehicles using video surveillance cameras (VAPI) #ERDF
-
Artifical intelligence for more precise diagnostics (AI4DIAG) #ESIF
-
Smart non-contact phenotyping of raspberries and quinces using machine learning methods, hyperspectral and 3D images (AKFen) #ESIF
-
A Deep Learning Approach for Osteoporosis Identification using Cone-beam Computed Tomography (OSTAK) #ESIF
Recent publications
- Sudars, K., Jasko, J., Namatevs I., Ozola L., Badaukis, N. (2020). Dataset of annotated food crops and weed images for robotic computer vision control, Data in Brief, 31. doi:10.1016/j.dib.2020.105833
- Sudars, Kaspars, Ivars Namatēvs, and Kaspars Ozols. 2022. "Improving Performance of the PRYSTINE Traffic Sign Classification by Using a Perturbation-Based Explainability Approach" Journal of Imaging 8, no. 2: 30. https://doi.org/10.3390/jimaging8020030
- Arturs Nikulins, Kaspars Sudars, Edgars Edelmers, Ivars Namatevs, Kaspars Ozols, Vitalijs Komasilovs, Aleksejs Zacepins, Armands Kviesis, Andreas Reinhardt. "Deep Learning for Wind and Solar Energy Forecasting in Hydrogen Production" Energies 17(5): pp.12. https://www.mdpi.com/1996-1073/17/5/1053