Land cover classification from satellite images is one of the main Earth observation (EO) tasks starting from the launch of first EO satellites. It is used for producing land cover maps showing areas covered by forests, agriculture areas, water basins, roads, buildings and other categories that are needed for the accounting of land resources. We have created an automatic classification technology which is unsupervised and highly robust (hereafter Dynland). Our working prototype allows users to classify multispectral geospatial images into land use and land cover classes. Dynland technology enables the use of machine learning methods in applications where it was not possible before due to the lack of sufficient training data. For others, lesser requirements on training data mean significantly lower cost and faster delivery of geospatial analytical results.
Dynland unsupervised classification technology for geospatial image analysis is on the overlap of two industries: Earth observation (EO) and machine learning (ML). EO capabilities are rising with 596 satellites devoted to Earth observation. EO data has become widely available through public investment in EU (Copernicus programme) and private investment in the USA (190 satellites in Planet Labs constellation alone). Daily optical imaging anywhere in the world is available. EO Data acquisition through unmanned aerial vehicles (UAV) like drones has become mainstream. UAV based EO data is already used in agriculture and will enter other industries with an integration of UAVs into shared airspace.
We have done an internal and third-party comparison of our clustering algorithm with most popular clustering and classification algorithms. Tests were done on both synthetic as well as real multispectral satellite data. Our algorithm outperformed other clustering algorithms in terms of accuracy of making proper clusters of spectrally close pixels related to one subclass of the data. Classification with Dynland is possible in circumstances where supervised algorithms fail due to a lack of sufficient training data.
Interviews with potential users of EO data reveal problems that we see as opportunities:
- collection of reliable training data in many applications is not only expensive but often impossible
- ground truth data is not shared among projects even in cases where it would add value
- building internal competence, purchasing expensive remote sensing software packages, hardware and training often outweigh the benefits
- there are inefficiencies in commercial EO satellite data pricing – minimum order sizes of 25km2 to 100km2 at 5-100 EUR/km2 is often too much for small, geographically or temporally scattered EO tasks.
We have started commercialization of Dynland that address the identified market problems. Our technology is generic enough to add value in most geospatial image classification tasks. Our priority applications are in classifying multispectral imagery in forestry, precise agriculture, insurance and land cover classification for public institutions. There is a significant demand for fully automated value-added services that do not require training data. Fully automated EO services are among the fastest-growing segments of EO industry with 38% CAGR.
Our technology provides a highly accurate classification of the objects on the image without the use of training data. It takes a satellite image (1), forms clusters of pixels, then assigns classes to clusters (2) and provides users with information about classes and their sizes (3).
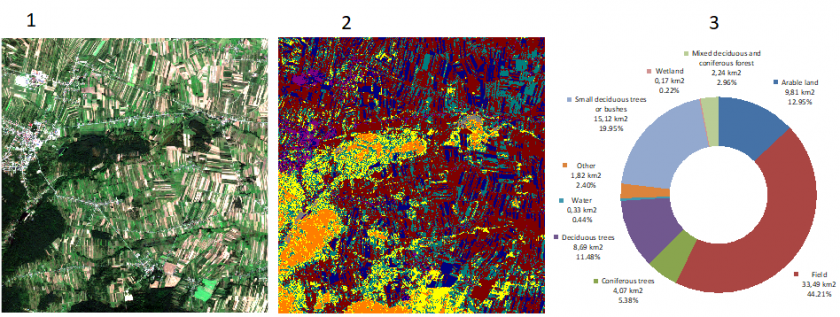
For a more technical description of the technology, please read our public whitepaper.
Information about the completed and ongoing projects related to Earth Observation can be found here.
Projects
- Sentinel for confidence in outdated maps: SentiMap
- Remote sensing based system for forest risk factor monitoring (Forest Risk) #ESIF
-
Satellite remote sensing- based forest stock estimation technology (WoodStock) #ESIF
-
Dynamic land use monitoring by fusion of satellite data (DynLand) #ESA
- Cyber-physical systems, ontologies and biophotonics for safe&smart city and society (GUDPILS) #SRP (VPP)
- Identification of tree species in Latvian forests (TrIdent) #SRP (VPP)
-
Automated identification of mires and peatlands using multi-temporal satellite data (MireClass) #ESA
-
Dynamic land use monitoring (NevKlas) #ESIF
- High precision synchronous timing in widely distributed scientific instrumentation (DaLaS) #ESIF
- Innovative technologies for acquisition and processing of biomedical images (InBiT) #ESIF
- Multi-model development technology for .NET application projects (MEDUS) #ESIF
Publications
- Martins Pukitis, Ints Mednieks. "Classification of satellite images using Dynland technology" Proc. SPIE 12786, Ninth International Conference on Remote Sensing and Geoinformation of the Environment (RSCy2023), 127860E (21 September 2023); https://doi.org/10.1117/12.2681896
- Andris Skromulis, Juris Breidaks, Mārtiņš Puķītis. 2023. "Wetland Change Detection Using Sentinel-2 in the Part of Latvia" Environment. Technology. Resources. Proceedings of the International Scientific and Practical Conference. https://doi.org/10.17770/etr2023vol1.7305
- Linda Gulbe, Juris Zarins, Ints Mednieks, “Automated delineation of microstands in hemiboreal mixed forests using stereo GeoEye-1 data”. MPDI Open Access Journals, Remote Sens. 18 March 2022. https://www.mdpi.com/2072-4292/14/6/1471.
- Sinica-Sinavskis J., Dinuls R., Zarins J., Mednieks I., Automatic tree species classification from Sentinel 2 images using deficient inventory data, 2020 17th Biennial Baltic Electronics Conference (BEC), Tallinn, Estonia, pp. 1-6, 2020.
- Romans Dinuls, Ints Mednieks. (2018). Nonparametric Classification of Satellite Images.ICoMS 2018 Proceedings of the 2018 International Conference on Mathematics and Statistics Pages 64-68, Porto, Portugal — July 15 - 17, 2018
- LORENCS A, MEDNIEKS I,SIŅICA-SIŅAVSKIS J. 2016 "Selection of Informative Bands for Classification of Hyperspectral Images Based on Entropy", Proc. of BEC2016, the 15th Biennial Conference on Electronics and Embedded Systems. Tallinn, Estonia on October 3-5, 2016. pp. 135-138.
- A. Lorencs, I. Mednieks & J. Sinica-Sinavskis. Selection of informative hyperspectral band subsets based on entropy and correlation. International Journal of Remote Sensing, 39:20, pp. 6931-6948, 2018. DOI: 10.1080/01431161.2018.1468107
- LORENCS, A., MEDNIEKS, I., SINICA-SINAVSKIS, J., 2015. Classification of Multisensor Images with Different Spatial Resolution, Electronics and Electrical Engineering, Vol. 21, No. 5, pp.81-85
- LORENCS, A., MEDNIEKS, I., SINICA-SINAVSKIS, J. 2014. Simplified Classification of Multispectral Image Fragments. Electronics and Electrical Engineering. Kaunas: Technologija, 20(6), pp. 136–139.
- A.Lorencs, J.Sinica-Sinavskis, “Analysis of two-stage Bayes classifiers construction method: 2-dimensional case,” Automatic Control and Computer Sciences, September 2013, Vol. 47, No. 5, pp. 254-266, 2013.
- G.Erins, A.Lorencs, I.Mednieks, J.Sinica-Sinavskis. “Tree Species Classification in Mixed Baltic Forest,” Proceedings of 3rd Workshop on Hyperspectral Image and Signal Processing: Evolution in Remote Sensing (WHISPERS), 2011, pp.1-4.
- R. Dinuls, A. Lorencs, I. Mednieks. "Using Consolidated Covariance Image for Discrimination of Habitats," Proceedings of the 13th Biennial Baltic Electronics Conference, Tallinn, Estonia, pp.299-302, 2012.
- I.Mednieks. "A Method for Correction of Rural Multispectral Aerial Image Mosaics." Proceedings of the 13th Biennial Baltic Electronics Conference, Tallinn, pp.295-298, 2012.
- A. Lorencs, Yu. Sinitsa-Sinyavskis. "A two-stage method for building classifiers," Automatic Control and Computer Sciences, September 2012, Volume 46, Issue 5, pp. 214-222.
- A. Lorencs, I. Mednieks, J. Sinica-Sinavskis, “Design problems of tree species classifiers for multispectral images,” Automatic Control and Computer Sciences, Vol. 45, No. 2, pp. 61-69, 2011.
- R. Dinuls, A. Lorencs, I. Mednieks. “Performance Comparison of Methods for Tree Species Classification in Multispectral Images,” Electronics and Electrical Engineering. Kaunas: Technologija, 2011, No.5(111), pp. 119–122.
- R. Dinuls, G. Erins, A. Lorencs, I. Mednieks, and J. Sinica-Sinavskis, “Tree species identification in mixed Baltic forest using LiDAR and multispectral data,” IEEE J. Sel. Topics Appl. Earth Observ. Remote Sens., vol. 5, no. 2, pp. 594–603, 2012.
- LORENCS, A., Sinica-Sinavskis, j., Jakovels, d., Mednieks, i., 2016. Melanoma-Nevus Discrimination Based on Image Statistics in Few Spectral Channels, Electronics and Electrical Engineering (Elektronika ir Elektrotechnika, Vol. 22, No2, pp. 66-72
- A.A.Lorencs. Multidimensional Observation Plans Inducing Nondegeneracy of Information Matrixes of Regression Models. Automatic Control and Computer Sciences, 2010, Vol.44, No.2, pp.69-77.
- A. Lorencs, J. Sinica-Sinavskis. One method of image processing and its numerical analysis. Electronics and Electrical Engineering. Kaunas; Technologija, 2010. No.7 (103), pp.25-29.
- I.Mednieks. Morphology-Based Approach to Detection of Free Form Line Objects in Grayscale Images. Electronics and Electrical Engineering - Kaunas: Technologija, 2010, No. 8(104), pp. 27-30.
- A.Lorencs, I.Mednieks, J.Sinica-Sinavskis. “Fast Object Detection in Digital Grayscale Images”, Proceedings of the Latvian Academy of Sciences. Section B., 2009, Vol.63, No.3, pp.116-124.
- I.Mednieks, A.Skageris. “Real Time Image Processing for Object Detection”, “Electronics and Electrical Engineering”, Kaunas: Technologija, 2009, No.4(92), p.33-36.
- A.Lorencs, I.Mednieks, J.Sinica-Sinavskis. Biomedical Image Processing Based on Regression Models. NBC - 14th Nordic-Baltic Conference on Biomedical Engineering and Medical Physics, Riga, June 16-20, 2008. IFMBE Proceedings, Vol.20, pp. 536-539.
- I.Mednieks. Object detection in grayscale images based on covariance features. Proceedings of ICSES 2008, Krakow, September 2008, pp. 205-209